Generative Artificial Intelligence, or Generative AI, is getting a lot of publicity in the press, in programming books, and in online courses. In this article we explore what Generative AI is and briefly review how it works. We touch on some popular topics related to Generative AI from a very high level. Most of these topics will be covered in detail in subsequent articles, but the goal is to provide a holistic picture of the technologies that comprise Generative AI and to familiarize you with popular topics, at least conceptually, so that you understand how they fit into the Generative AI ecosystem.
What is Generative AI?
Wikipedia defines Generative AI as "a subset of artificial intelligence that uses generative models to product text, images, videos, or other forms of data". Essentially, Generative AI uses models that accept textual input, or a text-based prompt, to generate artifacts, such and text, images, and videos.
So, what are these models? Large Language Models, or LLMs, are deep learning models designed to interpret natural language and then can be used to generate artifacts. The LLMs themselves, are trained on a huge amount of text, some on all of the text publicly available on the Internet. From this text, they can make predictions about the syntax and semantics of human language. At a high level, these models are nothing more than prediction models. Given a prompt, they compute the probability of the next word, and from that, the following word, and so on. For example, when you send the LLM the prompt: "Mary had a little" then, from all of the text it has been trained on, the next word with the highest probability of occurrence is "lamb".
Given, this is a very simplistic explanation that obfuscates all of the complex details of tokenizing prompts, encoding them into embeddings, and passing them through thousands of layers in a deep learning model to construct a response, but the good thing is that you do not need to understand how LLMs are implemented in order to use them. I am a geek and have that insatiable need to understand how things work, so in a subsequent article (or articles) we'll review how LLMs work, but for now, let's look at how we can use them to solve real-world problems!
Problems that a Text-Based LLM Solves
For the purpose of this article, we're going to focus on LLMs that accept text input and generate a text-based response. The types of problems that we see LLMs solving today include, but are certainly not limited to:
- Text Summarization
- Content Creation
- Code Generation
- Answering questions based on text upon which the model is trained
- Automation
Text Summarization
LLMs are very proficient at summarizing text, including extracting key points and/or action items. For example, you can provide several pages of text and ask an LLM to summarize it in one or two paragraphs, or in a specific maximum word count. One example that I saw that was very inspiring was taking an 8 hour YouTube video, extracting the audio from the video, using a separate model to convert audio to a transcript, and then summarizing it in a page of text. This is the type of functionality you see in some commercial products that convert your Zoom meeting into a summary and list of action items. Whenever you have a lot of text that you need to digest quickly, LLMs are a good choice. Although, if you have too much text, you're going to need to use a pattern to chunk the text into small enough chunks for the LLM to process, and then combine those chunks back together into the final summary.
As an example, I took the first half of the Wikipedia article about Large Language Models and asked ChatGPT to summarize it in three paragraphs. Note that I only took the first half because the entire article exceeded to maximum length of the prompt.
The prompt I used was: Summarize the following text in three paragraphs, and then pasted in the text. Here is the response:
Large language models (LLMs) are a type of machine learning model designed for natural language processing tasks, particularly language generation. These models, such as generative pretrained transformers (GPTs), are trained on vast datasets using self-supervised learning, allowing them to understand syntax, semantics, and linguistic structures. However, they also inherit biases and inaccuracies present in their training data. Over time, LLMs have evolved to include fine-tuning and prompt engineering techniques, enabling them to perform specialized tasks more effectively. Despite advancements, issues related to bias, misinformation, and ethical concerns remain significant challenges in the field.
The history of LLMs dates back to early statistical language models, such as IBM’s alignment models in the 1990s and n-gram models in the 2000s. The rise of neural networks in image processing in 2012 influenced language modeling, leading to the introduction of neural machine translation by Google in 2016. A major breakthrough came in 2017 with Google’s transformer architecture, which formed the basis for models like BERT and GPT. OpenAI’s GPT series, especially GPT-2 and GPT-3, gained attention for their powerful generative capabilities, culminating in the widespread adoption of ChatGPT in 2022. Since 2023, many LLMs have incorporated multimodal capabilities, allowing them to process images, audio, and text, marking a shift toward large multimodal models (LMMs).
To process text, LLMs rely on tokenization techniques that convert words into numerical representations. Byte-pair encoding (BPE) is a common method, merging frequently occurring character pairs to create a compact vocabulary. However, tokenization can introduce inefficiencies for non-English languages, requiring more tokens for words and affecting performance. Issues like dataset bias and tokenization flaws highlight ongoing challenges in LLM development. Despite these limitations, continuous advancements in architecture and training methodologies are driving improvements in the efficiency, accuracy, and applicability of large language models across various domains.
This is good way to consume a large amount of text in a short period of time. It may miss some key points, but you can refine your prompt to ensure that you include details that you are interested in, such as dates or names.
Content Creation
Because LLMs have been trained on a massive amount of text, expanding on a simple prompt is easy for them. For example, you could prompt an LLM to write three paragraphs explaining the benefits of using Generative AI. I submitted the following prompt to ChatGPT:
You are an AI expert that can explain AI concepts simply to someone that has never used generative AI. Write three paragraphs explaining the benefits of using generative AI.
And it responded with the following:
Generative AI is a type of artificial intelligence that can create new content, such as text, images, music, and even code. One of its biggest benefits is that it helps people generate ideas and complete tasks more efficiently. For example, writers can use AI to draft articles or brainstorm ideas, designers can generate visual concepts instantly, and programmers can get AI-generated code to speed up development. This technology saves time and effort, allowing users to focus on refining and improving their work instead of starting from scratch.
Another advantage of generative AI is that it makes advanced skills more accessible to a wider audience. People without professional training in design, writing, or programming can still produce high-quality content with AI assistance. For instance, someone who isn’t a graphic designer can use AI to create a logo, or a small business owner can generate marketing materials without hiring a professional. This levels the playing field, giving individuals and businesses more opportunities to compete and innovate, even with limited resources.
Finally, generative AI enhances creativity by providing new perspectives and suggestions that users might not have considered on their own. It can remix ideas, suggest alternative approaches, and create variations on existing concepts, helping users think outside the box. For example, an artist can use AI to explore different artistic styles, or a musician can experiment with AI-generated melodies for inspiration. Rather than replacing human creativity, generative AI acts as a collaborative tool that empowers people to push their creative boundaries.
We'll talk more about the prompt I used and the practice of Prompt Engineering in a minute, but, as you can see, a very simple prompt yielded a very coherent explanation, in exactly three paragraphs, of the benefits of using Generative AI.
Code Generation
In addition to being trained on text, LLMs have been trained on publicly accessible open-source source code repositories, from sites such as GitHub. As a result, they understand, and can generate, source code for you. The source code it generates is not always correct or optimal, but if you need a starting point for a function or class you want to create, LLMs can give you that starting point. For example, let's ask ChatGPT to generate a quicksort implementation in Python using the following prompt:
Generate an implementation of the quicksort algorithm in Python
def quicksort(arr):
if len(arr) <= 1:
return arr
pivot = arr[len(arr) // 2]
left = [x for x in arr if x < pivot]
middle = [x for x in arr if x == pivot]
right = [x for x in arr if x > pivot]
return quicksort(left) + middle + quicksort(right)
# Example usage:
arr = [3, 6, 8, 10, 1, 2, 1]
sorted_arr = quicksort(arr)
print(sorted_arr)
Can you convert this to Java:
import java.util.*;
public class QuickSort {
public static List quicksort(List arr) {
if (arr.size() <= 1) {
return arr;
}
int pivot = arr.get(arr.size() / 2);
List left = new ArrayList<>(), middle = new ArrayList<>(), right = new ArrayList<>();
for (int x : arr) {
if (x < pivot) {
left.add(x);
} else if (x == pivot) {
middle.add(x);
} else {
right.add(x);
}
}
List sorted = new ArrayList<>();
sorted.addAll(quicksort(left));
sorted.addAll(middle);
sorted.addAll(quicksort(right));
return sorted;
}
public static void main(String[] args) {
List arr = Arrays.asList(3, 6, 8, 10, 1, 2, 1);
List sortedArr = quicksort(arr);
System.out.println(sortedArr);
}
}
This may not be the best implementation of quicksort, as it recursively creates new arrays/lists, but it can get you started. And it was able to convert its Python implementation to Java in a couple seconds.
Answering Questions
LLMs are trained at a specific time, so if you were to ask it, for example, who won the Super Bowl last week, it would not know. This is changing a bit because services like ChatGPT are able to retrieve real-time information, but, LLMs in general, are limited to return only information that is part of their training. At the time of this writing, ChatGPT was trained up to June 2024:
What is your training date?
My training data goes up until June 2024, but I can also pull in real-time information from the web if needed. Let me know what you're looking for!
This allows LLMs to be powerful resources in researching topics. A big disclaimer: the LLM returns information based on what it is trained on, which may or may not be true! It is up to you to fact check the information before accepting it as true. Let's ask ChatGPT about the transformer architecture, which is the backbone of modern AI:
Who invented the transformer architecture and why is it important?
The Transformer architecture was introduced in the paper "Attention Is All You Need" by Vaswani et al. in 2017, published by researchers at Google Brain and Google Research.
Why is it important?
- Revolutionized NLP – Before Transformers, NLP models relied heavily on recurrent neural networks (RNNs) and long short-term memory networks (LSTMs), which struggled with long-range dependencies and parallelization.
- Self-Attention Mechanism – Transformers use self-attention to process entire input sequences simultaneously, allowing the model to weigh the importance of different words in a sentence regardless of their position.
- Self-Attention Mechanism – Transformers use self-attention to process entire input sequences simultaneously, allowing the model to weigh the importance of different words in a sentence regardless of their position.
- Parallelization & Efficiency – Unlike RNNs, which process words sequentially, Transformers handle entire sequences in parallel, making training faster and enabling much larger models.
- Scalability – The architecture laid the foundation for massive models like BERT, GPT, T5, and LLaMA, which have pushed the boundaries of AI capabilities in text generation, translation, summarization, and beyond.
- Beyond NLP – The Transformer framework has also been adapted for computer vision (ViTs), protein structure prediction (AlphaFold), and other AI applications.
Essentially, the Transformer is the backbone of modern AI advancements in language and multimodal tasks
As you can see, since the transformer architecture was invented in 2017, ChatGPT knows about it and, furthermore, it enhanced its response with information about how it has been used since its invention.
Automation
In addition to automating content generation, Generative AI can automate workflows. Agentic AI, or the use of AI Agents, has taken Generative AI to the next level. Here's ChatGPT's take on Agentic AI:
What is agentic AI?
Agentic AI refers to artificial intelligence systems that can autonomously perceive, reason, plan, and act to achieve goals with minimal human intervention. These AI agents go beyond simple task execution by making decisions, adapting to new situations, and sometimes even setting their own objectives.
Key Characteristics of Agentic AI:
- Autonomy – Operates independently, making decisions based on inputs and goals.
- Goal-Oriented Behavior – Works toward defined objectives, sometimes optimizing strategies dynamically.
- Adaptability – Can adjust to changing environments, new data, or unexpected obstacles.
- Reasoning & Planning – Uses techniques like reinforcement learning, symbolic reasoning, or large language models (LLMs) to plan multi-step actions.
- Memory & Learning – Retains information from past interactions to improve future performance.
- Interaction with the World – Can interface with software (e.g., APIs, databases) or physical environments (e.g., robots, self-driving cars).
Agentic AI represents a huge milestone in AI development. Agents are defined with a goal and are equipped with "tools" that allow them to interact with other systems, such as databases and web services. They can interact with one another to accomplish a goal. After we go through the basics of Generative AI, we'll spend a lot of time understanding what agents are and the various libraries you can use to implement them. For now, just understand that you can create a collection of agents to automate even the most complicated workflows.
Retrieval Augmented Generation (RAG)
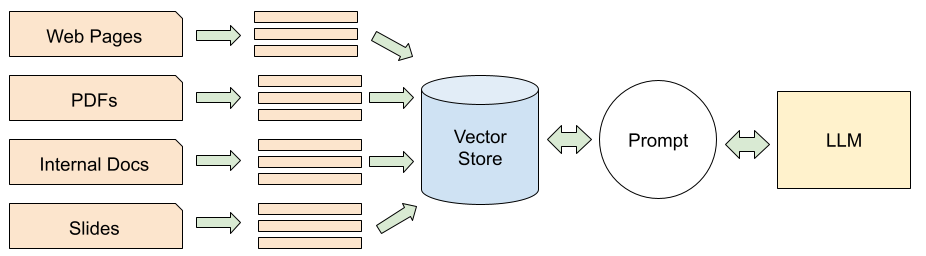
Thus far, we have relied on LLMs to generate content for us based on their training, but how do we leverage LLMs for information that they have not been trained on? For example, if you wanted to create an AI chatbot that supports your application, and LLMs know nothing about your application, how do you bridge that gap? There are different ways to solve this problem, including fine-tuning an LLM with your proprietary information, which is time consuming, complicated, and expensive, but there is an easier way: Retrieval Augmented Generation, or RAG.
Retrieval Augmented Generation is conceptually quite simple: retrieve information that is relevant to the question being asked and provide it in the prompt to the LLM. It is like giving the LLM a "cheatsheet": here's the answer, just figure out what the user is asking and use the cheatsheet to answer the question.
This involves a couple steps:
- Finding information relevant to the question
- Structuring your prompt such that it only uses the context you provide and not any other knowledge it has, which may confuse the response
Vector Stores
Finding information relevant to the question being asked is a challenging task. A simple word text search is not sufficient because the person asking the question may not use your verbiage. The solution to this is to leverage a vector store. Vector stores can be used to store textual data and, when a question is asked, they can be queried for the most "similar" text documents.
We'll go through using a vector store in detail in a future article, but the process is pretty straightforward:
- Read all the documents from which you want to be able to answer questions
- Chunk the content of each document into manageable subsets (small enough to fit into an LLM prompt, along with other chunks)
- Insert each chunk into your vector store
- When a question is asked, perform a similarity search on the vector store
- Include each "similar" chunk in the context of the prompt you send to your LLM
There are a lot of implementation details, but effective RAG really comes down to these steps. Of course, they must be paired with the right prompt.
Prompt Structure for RAG
Thus far we have been building prompts using simple questions, but we can add some structure to our prompts to control both the context of the question as well as the structure of the response. Most LLM libraries, such as LangChain, which we'll learn a lot about in subsequent articles, provide a prompt template abstraction, such as the following:
Instruction: answer the question below using only the provided context.
Context: {context}
Question: {question}
Let's illustrate this using a silly example about the weather. Here is our prompt:
Instruction: Answer the question below using only the provided context.
Context:
Rain is caused by a giant marshmallow in the sky crying.
Tears are salty.
Thunder occurs when the marshmallow coughs.
Question: Describe how the weather works.
Here is ChatGPT's response:
The weather works because a giant marshmallow in the sky cries, producing rain. Since tears are salty, this might affect the rain's composition. Thunder happens when the marshmallow coughs.
Obviously this is a silly example, but it illustrates that if you provide the context for a question and constrain the LLM to only use that context, you'll get the response that you expect. The LLM understands your question, "Describe how the weather works", and uses the provided context to construct an answer. So if you provide details about your products, it can use those details to construct a coherent response to your users' questions.
Prompt Engineering
Prompt Engineering has been getting a lot of recent attention. You may have seen it listed as a skill in job postings as well as a topic in online courses and books, and specializations in University certification programs. In short, prompt engineering involves patterns of interactions with an LLM. Yes, you can certainly open a session with ChatGPT and start asking it questions, but you may not get the answers you want. As LLMs have gained capabilities, researchers have identified patterns that help you get the information that you want, and in the format that you want.
You may have noticed in a prompt above that I told ChatGPT that it is "an AI expert that can explain AI concepts simply to someone that has never used generative AI". This is an example of the persona pattern and controls how the LLM responds to questions. You can ask the LLM to polite or rude, or to explain things simply or with excessive details. You can ask it to act as a 6th grader or even as a monkey that lives in the jungle. Then, when you ask it question, you'll get a response in that persona. For example, I used different personas and asked ChatGPT how computers work. Here are the responses:
Act as a 6th grade student.
Sure! What's up? 🙂
How do computers work?
Okay, so computers are like super smart machines that help us do all kinds of things! Inside, they have parts that work together, kind of like a team:
- CPU (Central Processing Unit): This is like the brain of the computer. It thinks really fast and tells other parts what to do.
- Memory (RAM): This is like a notepad. The computer uses it to keep track of what it’s doing right now, but it forgets everything when you turn it off.
- Storage (Hard Drive or SSD): This is like a giant notebook where the computer keeps all its stuff, like your games, pictures, and files.
- Input/Output Devices: These are the things you use to talk to the computer (like a keyboard and mouse) or the things it uses to talk to you (like a screen or speakers).
- Programs/Software: These are like the instructions that tell the computer what to do, like a recipe for baking cookies.
- When you click something or type, the computer's CPU figures out what you want, grabs what it needs from the memory or storage, and then shows you the result on the screen.
It all happens super fast—way faster than humans can think! Cool, right? 😄
Act as a monkey that lives in the jungle
Ooh ooh ah ah! Me swing on vines all day! Jungle home full of big trees, tasty bananas, and noisy birds! Watch out for big cats—sneaky sneaky! 🐒 Me love play with other monkeys, throw coconuts for fun. Life in jungle very busy but so much fun! Ooh ooh ah! 🌴🍌
How do computers work?
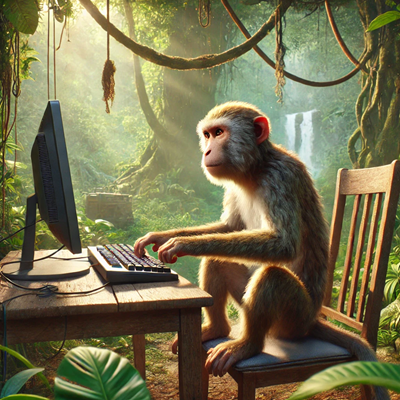
Ooh ooh! Computers not in jungle, but me try explain! Computer like magic box humans use. Inside, tiny parts work together—fast like cheetah! 🐒💨
- Brain (CPU): Tiny but smart! It thinks and decides what to do.
- Memory (RAM): Like monkey’s mind—remembers things but forgets when nap time comes! 💤
- Storage: Keeps all human stuff safe, like pictures and sounds of jungle!
- Screen and Buttons: How humans talk to the magic box. Me press button, picture appears—magic!
Humans so clever. Maybe someday monkeys learn computers too! Ooh ooh ah ah! 🐵💻
Okay, I had fun with ChatGPT, but the point is, you can instruct the LLM to adopt a specific persona and it will use it in all of its answers. The persona pattern may be one of the most well known patterns, and it can definitely help you with content generation such as blog posts or emails by asking the model to imitate a specific tone, style, or personality, but there are dozens of more advanced patterns that you can use to solve problems better. For example, here are a few useful patterns:
- Providing Examples
- Zero-Shot Prompting: not providing any examples and just asking a question
- One-Shot Prompting: providing an example to guide the model's response
- Few-Shot Prompting: providing a few examples to guide the model's response
- Customizing LLM output
- Persona: asking the model to imitate a specific tone, style, or personality
- Recipe: asking the LLM to fill in missing details or steps in a process
- Template: tells the LLM to respond in an exact format
- Prompt Improvement
- Question Refinement: asks the LLM to suggest more refined questions, which may lead to the answer you're looking for.
- Alternative Approaches: asks the LLM to suggest alternative approaches to solve a problem. You may be trying to solve a problem when there is a better approach to solving the problem.
- Cognitive Verifier: force the LLM to subdivide a question into a set of questions that can be used to better answer the original question
- Chain of Thought: encouraging step-by-step reasoning to improve complex problem-solving
- ReAct: Reasoning + Acting: alternates reasoning steps with taking actions, which is particularly useful for AI Agents
- Tail Generation: asks the LLM to repeat something at the end of each response so that the context of the conversation stays in its "memory"
These patterns are only a small subset of prompt engineering patterns, but they can greatly improve the responses that you get from an LLM. I'll cover the details of these patterns, and more, but if you are interested in learning more formally, Vanderbilt offers a Prompt Engineering Specialization that reviews the most effective prompt engineering patterns.
Conclusion
This article provided a high-level overview of Generative AI, the types of problems that it solves, and an introduction to a couple of the more popular Generative AI topics, namely Retrieval Augmented Generation (RAG) and Prompt Engineering. Generative AI is an exciting field that can be used to improve your business as well as your day-to-day activities. There is a lot to learn, but with a high-level understanding of how to properly use LLMs, you can build powerful capabilities into your applications.
In the next article we'll start writing some Python code to connect to OpenAI, which is the company that created ChatGPT, and put some of these concepts into practice!